Processors that mimic the human brain
could be the next big disruption in computing
How long can the industry rely on Moore's
Law? Today's computers are rather too good at calculating stuff and can achieve
anything that can be reduced to a numerical problem. But complex problems,
which need a good amount of reasoning or those that need so-called 'intuition/
require too much programming and hence too much processing and power too. Just
stuffing more transistors into smaller chips will take us nowhere. So, what
next?

Today's
computers are rather too good at calculating stuff and can achieve anything
that can be reduced to a numerical problem.
Seeking to move to the next new frontier of
computing, research teams across the world are trying to move away from
traditional chip designing methods and radically redesign memory, computation
and communication circuitry based on how the neurons and synapses of the brain
work. This will be a big leap in artificial intelligence, eventually resulting
in self-learning computers that will be able to understand and adapt themselves
to changes, complete tasks without routine programming and work around failures
too. Such self-learning computers are commonly dubbed as 'neuromorphic' as they
mimic the human brain. Here, we look at some of the significant strides in this
direction.
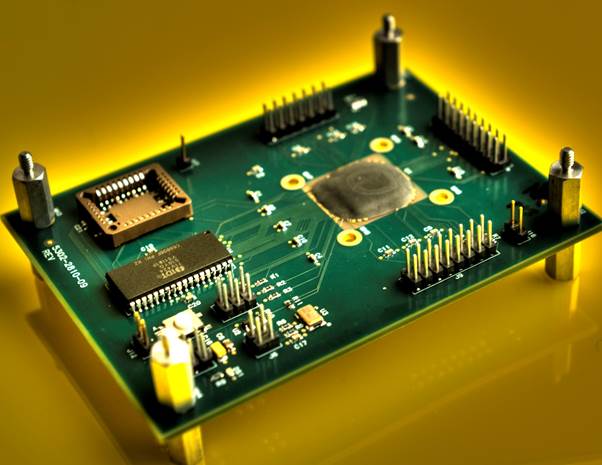
Seeking
to move to the next new frontier of computing, research teams across the world
are trying to move away from traditional chip designing methods and radically
redesign memory, computation and communication circuitry based on how the
neurons and synapses of the brain work.
Mimicking the mammalian brain in function, size and power
consumption
One of the largest and oldest projects in
this direction is the DARPA sponsored Systems of Neuromorphic Adaptive Plastic
Scalable Electronics (SyNAPSE), which is contracted mainly to IBM and HRL,
along with some US-based universities. The goal of the project is to build a
processor Phicsor that imitates a mammal's brain in function, size and power
consumption. Specifically, "It should recreate 10 billion neurons, 100
trillion synapses, consume one kilowatt and occupy less than two litres of
space." Since it started in 2008, the project has seen some interesting
results.
The first breakthrough came in 2011, when
IBM revealed two working prototypes of neurosynaptic chips.
Both the cores were fabricated in 45nm
silicon on insulator (SOI) complementary metal oxide semiconductor (CMOS) and
contained 256 neurons. One core had 262,144 programmable synapses while the
other had 65,536 learning synapses. Then came the Brain Wall —a visualisation
tool that allows researchers to view neuron activation states in a large-scale
neural network and observe patterns of neural activity as they move across the
network. It helps visualise supercomputer simulations as well as activities
within a neurosynaptic core.
Meanwhile, in 2012, IBM demonstrated a
computing system called TrueNorth that simulated 530 billion neurons and 100
trillion synapses, running on the world's second-fastest operating
supercomputer. TrueNorth was supported by Compass — a multi-threaded, massively
parallel functional simulator and a parallel compiler that maps a network of
long-distance pathways in the macaque monkey brain to TrueNorth.
Last year, they had more updates. IBM
revealed that the chips are radically different from the current Von Neumann
architecture based ones. The new model works with multiple low-power processor
cores working in parallel. Each neurosynaptic core has its own memory
(synapses), a processor (neuron) and communication conduit (axon). By operating
these suitably, one can achieve recognition and other sensing capabilities
similar to the brain. IBM also revealed a software ecosystem that taps the
power of such cores, notably a simulator that can run a virtual network of
neuro-synaptic cores for testing and research purposes.
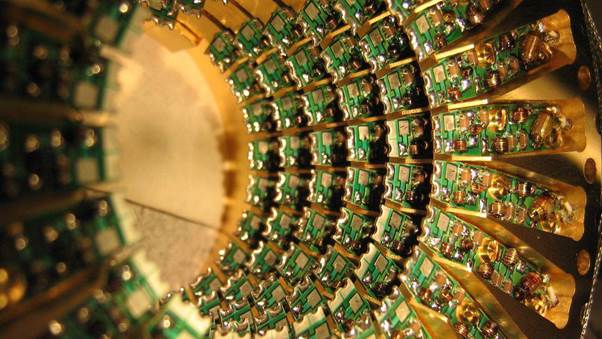
Last
year, they had more updates. IBM revealed that the chips are radically
different from the current Von Neumann architecture based ones.