Processors that mimic the human brain
could be the next big disruption in computing
The neuro-morphic processor constructed by
them consists of a 20cm-diameter silicon wafer containing an array of
identical, tightly-connected chips, with mixed-signal circuitry. While the
simulated neurons are analogue, the synaptic weights and inter-chip
communication are digital. Each wafer contains 48 reticles, each of which in
turn contains eight high-input-count analogue neural network (HICANN) chips,
making a total of 384 identical chips per wafer. Each 5x10 mm2 HI-CANN chip
contains an analogue neural core (ANC) as the central functional block, along
with supporting circuitry. In the current setup, each of the 384 chips
implements 128,000 synapses and up to 512 spiking neurons, totalling to
approximately 200,000 neurons and 49 million synapses per wafer.
Such a highly parallel chip design with
configurable electronic neurons helps the team to understand the dependencies,
synchronisation and communication among neurons and synapses, so the knowledge
can later be applied to computing.
Super chip for prosthetic limbs
A team at Stanford University, headed by
Kwabena Boahen, has also developed brain-inspired microchips, details of which
were revealed in an IEEE conference earlier this year. The team has used 16
custom-designed 'Neurocore' chips to develop a circuit board called the
'Neurogrid' that can simulate one million neurons and billions of synaptic
connections. The Neurogrid is 9000 times faster than today's personal
computers, and is about 100,000 times more energy-efficient than a PC-based
simulation of a million neurons. According to the team's estimates, a PC
consumes 40,000 times more power than a Neurogrid.
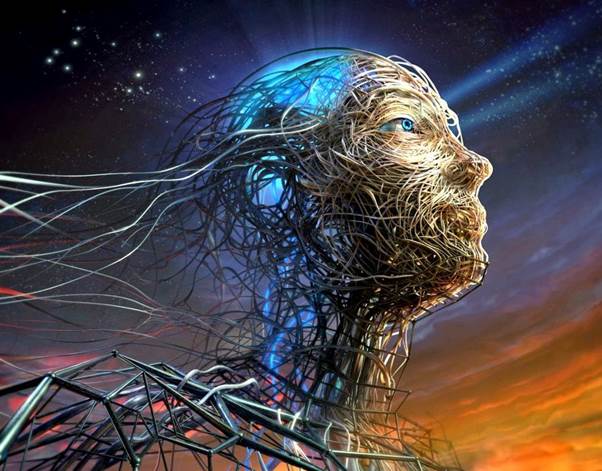
A
team at Stanford University, headed by Kwabena Boahen, has also developed
brain-inspired microchips, details of which were revealed in an IEEE conference
earlier this year.
Such speed and low-power characteristics of
the Neurogrid make it an ideal platform to model and understand the functioning
of the brain. Moreover, modified Neurocore chips can also be used for other
nature-mimicking functions, such as say, to control prosthetic limbs. This is
considered one of the most promising applications of the Neurogrid, and Boahen
is working very closely with other teams at Stanford University to achieve
this.
Comparing the Neurogrid to other similar
projects, such as SyNAPSE and Brain Scales, Boahen writes in his IEEE paper:
"Each of these research teams has made different technical choices, such
as whether to dedicate each hardware circuit to modelling a single neural
element (e.g., a single synapse) or several (e.g., by activating the hardware
circuit twice to model the effect of two active synapses). These choices have
resulted in different trade-offs in terms of capability and performance."
Boahen arrives at a single metric to calculate the total system cost including
the size of the chip, how many neurons it simulates and the power it consumes,
and uses it to show the Neurogrid as the most cost-effective way to simulate
neurons, in keeping with his goal of creating a system affordable enough to be
widely used in research.
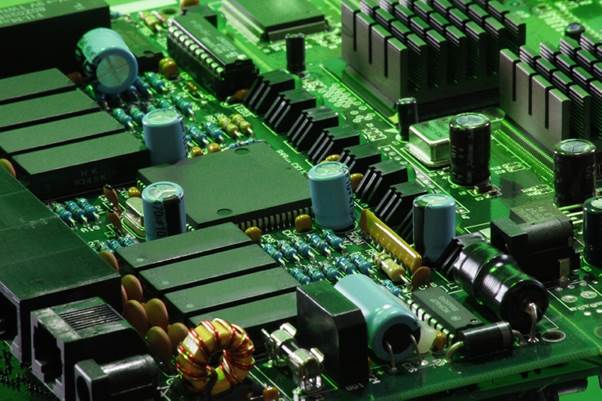
Boahen
arrives at a single metric to calculate the total system cost including the
size of the chip, how many neurons it simulates and the power it consumes, and
uses it to show the Neurogrid as the most cost-effective way to simulate
neurons.
However, there are a few areas that the
team is seriously working on improving now. One, of course, is the cost.
Currently, the development of each Neurogrid uses a 15-year old fabrication
process and costs around $40,000. By using modern processes and by fabricating
chips in larger volumes, the cost could be brought down to $400 per
million-neuron board.

By
using modern processes and by fabricating chips in larger volumes, the cost
could be brought down to $400 per million-neuron board.